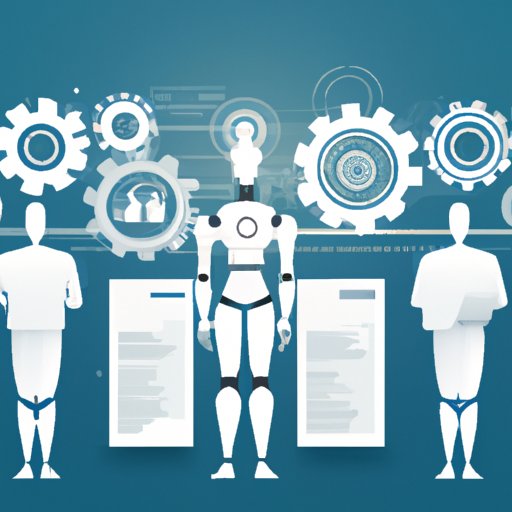
Understanding Predictive Analysis: Basics, Applications, and Future Trends
Predictive analysis is becoming increasingly important in various industries today. It is a powerful tool that enables businesses to make data-driven decisions and gain a competitive edge. In this article, we will explore the basics and importance of predictive analysis, the role of predictive analysis in business, showcase successful case studies, demystify machine learning, discuss challenges, and future trends of predictive analysis.
Start with the Basics: Define Predictive Analysis and Common Techniques
Predictive analysis is a technique used to analyze historical data and make predictions about future events or behaviors. It involves using various statistical and machine learning algorithms to identify patterns, trends, and relationships in the data.
There are several key terms associated with predictive analysis, including data mining, modeling, and scoring. Data mining involves discovering patterns or trends in large volumes of data. Modeling involves using mathematical equations to predict future outcomes. And scoring involves applying a set of rules to assign a score or probability to each potential outcome.
Some of the common techniques used in predictive analysis include regression analysis, decision trees, and neural networks. Regression analysis is a statistical method used to identify the relationship between two or more variables. Decision trees are a graphical representation of a set of decisions and their possible outcomes. And neural networks are a complex set of algorithms inspired by the workings of the human brain.
The Importance of Predictive Analysis: Identifying Trends, Patterns, and Potential Outcomes
Predictive analysis can help businesses identify trends, patterns, and potential outcomes of future events. It can provide insights into customer behaviors, market trends, and operational efficiencies. One of the advantages of using predictive analysis over traditional analytical methods is that it can help businesses make better-informed decisions.
There are several real-world examples of how predictive analysis has helped businesses make informed decisions. For example, the online retailer Amazon uses predictive analysis to make personalized product recommendations to its customers. By analyzing the browsing history and purchase patterns of its customers, Amazon can recommend products that are more likely to interest them.
Another successful example is the professional sports industry. Sports teams and leagues use predictive analysis to evaluate player performance, predict game outcomes, and identify strategic opportunities. By analyzing vast amounts of data, sports teams can gain a competitive edge and improve their chances of winning games.
The Role of Predictive Analysis in Business: Personalizing Customer Experiences and Increasing Profitability
Businesses use predictive analysis to personalize customer experiences and increase profitability. By analyzing customer data such as purchase history and browsing behavior, businesses can tailor their marketing campaigns, website design, and product offerings to better meet individual customer needs.
Predictive analysis can also help businesses increase profitability by identifying areas of inefficiency and waste. For example, manufacturers can use predictive analysis to optimize supply chain operations and reduce production costs. Restaurants can use predictive analysis to forecast demand and adjust staffing levels accordingly.
One real-world example of a business that has successfully implemented predictive analytics in its operations is UPS. UPS uses predictive analytics to optimize its delivery routes, reduce fuel consumption, and enhance customer service. By analyzing real-time data such as traffic patterns and weather conditions, UPS can make more informed decisions about how to allocate its resources.
Demystifying Machine Learning: Building Predictive Models
Machine learning is a subset of artificial intelligence that involves building predictive models based on data. Machine learning algorithms can be used to analyze vast amounts of data and identify patterns that humans might miss.
There are several key terms associated with machine learning, including supervised learning, unsupervised learning, and reinforcement learning. Supervised learning involves training a model on labeled data, meaning the data is already labeled with the correct outcome. Unsupervised learning involves training a model on unlabeled data and allowing it to find patterns on its own. And reinforcement learning involves training a model through trial and error.
Machine learning algorithms can be used to build predictive models that can identify patterns and predict future events. One real-world example is Netflix, which uses machine learning algorithms to recommend movies and TV shows to its users. By analyzing user behavior and preferences, Netflix can recommend content that is more likely to be of interest to its users.
Challenges of Predictive Analysis: Data Quality, Privacy, and Regulation
There are several challenges associated with predictive analysis, including data quality, privacy, and regulation. Data quality is a critical issue because predictive analysis depends on accurate data. If the data is incorrect or incomplete, the predictions will be unreliable.
Privacy is another challenge because predictive analysis often requires personal information, such as browsing history or credit card information. Businesses must be careful to protect the privacy of their customers and ensure that they comply with privacy regulations.
Regulation is another challenge because predictive analysis can have ethical implications. For example, there are concerns that predictive analysis could be used to discriminate against certain groups of people. Businesses must be aware of regulatory requirements and ensure that they operate within the law.
One real-world example of a business that has faced these challenges is Facebook. Facebook has been criticized for its use of personal data and for allowing advertisers to target specific groups of people based on race or ethnicity. In response, Facebook has implemented new policies to protect user privacy and prevent discrimination.
Future of Predictive Analysis: Emerging Technologies, Trends, and Innovations
The future of predictive analysis is bright, with emerging technologies such as AI, IoT, and big data analytics set to revolutionize the field. AI will enable predictive analysis to become more accurate and sophisticated, while IoT will generate more data for analysis. Big data analytics will allow businesses to store and analyze vast amounts of data.
There are several potential applications of these technologies, including predictive maintenance, fraud detection, and personalized medicine. Predictive maintenance involves using predictive analysis to identify potential equipment failures before they occur. Fraud detection involves using predictive analysis to identify suspicious activity and prevent fraud. And personalized medicine involves using predictive analysis to tailor medical treatments to individual patients.
However, these technologies also pose potential challenges, such as data security and privacy. Businesses must be aware of these challenges and ensure that they take appropriate steps to protect their data and comply with privacy regulations.
Conclusion
Predictive analysis is a powerful tool that can help businesses make data-driven decisions and gain a competitive edge. By identifying trends, patterns, and potential outcomes, businesses can make better-informed decisions and improve their overall performance. While there are challenges associated with predictive analysis, such as data quality and privacy, emerging technologies such as AI and IoT offer exciting opportunities for the future of the field.
Businesses that want to stay competitive in today’s fast-paced environment must start exploring predictive analysis and its potential applications. By leveraging the power of this technology, businesses can gain insights into customer behaviors, optimize operations, and enhance profitability.