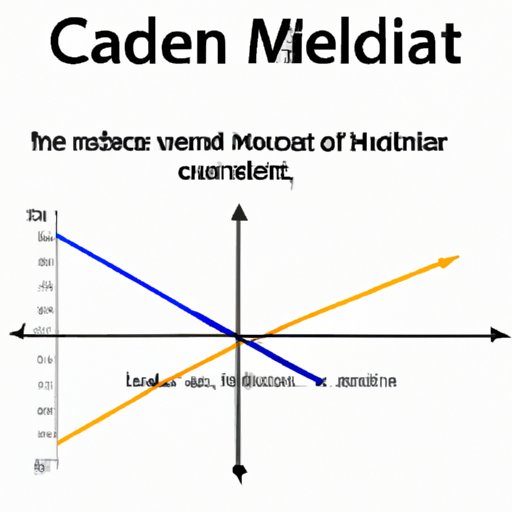
I. Introduction
Statistics is an essential part of data analysis across various fields, and one of the most important concepts is central tendency. When describing a set of data, we want to know where the data cluster around and how spread out they are. The central tendency measures help us describe the midpoint or center of the data. This article will focus on the median, one of the three measures of central tendency, and explain everything you need to know to understand and calculate it for your data set.
II. Understanding Median
The median is simply the number in the middle of a set of numbers. This central tendency measure is useful when there are extreme values, and we want to know the typical or middle value ignoring any outliers. We can only calculate the median for ordinal or interval/ratio data with an odd number of values without doing any calculations.
The median is an important measure of central tendency because it is less sensitive to extreme values or outliers than the mean. For example, if we have a set of data where half of the values are ten, and the other half is 100, the mean will be 55, which is not a representative value of either group. However, the median will be 10, which is the value that represents half of the data.
To compute the median for datasets with a large number of values, we sort the data and pick the middle value. The middle value is simply the value that has exactly half of the data above it and half below it.
III. A Step-by-Step Guide to Calculating the Median of a Data Set
To calculate the median, follow these steps:
A. Sorting the data set: First, we need to sort the data set in ascending or descending order.
B. Identifying the middle-most value: Next, we identify the middle value in the sorted dataset. The middle values depend on whether we have an odd or even number of values in the dataset. If we have an odd number of values, the median is the middle value. If we have an even number of values, we take the average of the two middle values.
C. Calculation of median: After identifying the middle value, we can conclude the median is simply that value. If it is the average of two values, we report that the median of the data set is the average of those two values.
IV. Examples of Real-Life Applications of Median Along with Its Calculation
The median has countless real-life applications, and here are some examples along with their calculations:
A. Median Income: Median income is the value of income that divides a population into two halves- those who earn more than the median and those who earn less than the median. For example, suppose we have the following incomes: $20,000, $50,000, $30,000, $46,000, $70,000, $12,000, $60,000, $100,000, and $40,000. After sorting the values in ascending order, we have $12,000, $20,000, $30,000, $40,000, $46,000, $50,000, $60,000, $70,000, and $100,000. The median income is $46,000.
B. Median Age: Median age is the midpoint value that separates the younger and older halves of a population. For example, suppose we have the following ages of students in a class: 18, 19, 20, 22, 23, 24, 25. After sorting them in ascending order, we have 18, 19, 20, 22, 23, 24, and 25. The median age is 22.
C. Median Household Size: The median household size is the value separating the larger and smaller halves of the households in a population. For example, suppose we have the following household sizes: 1, 2, 3, 3, 4, 4, 4, 5, 6. After sorting them in ascending order, we have 1, 2, 3, 3, 4, 4, 4, 5, and 6. The median household size is 4.
V. Comparing Median with Other Measures of Central Tendency in Statistics
There are three measures of central tendency: mean, median, and mode, but the median is the only one that is appropriate for ordinal data. The comparison of the median with other measures of central tendency reveals some critical aspects.
A. Mean: Mean is the arithmetic average of the dataset and is sensitive to outliers. A small number of outliers can lead to a substantial shift in the mean, whereas the same outliers may have no effect on the median. Therefore, the mean is not a suitable measure of central tendency if the dataset contains outliers.
B. Mode: Mode is the most frequently occurring value or values in a dataset. The mode can be useful in nominal data analysis. Unlike the mean and median, the mode is not affected by outliers because it deals only with the most frequently occurring value in the dataset. However, there can be no mode or multiple modes in a dataset, making it an inappropriate measure of central tendency in those cases.
VI. Common misconceptions related to Median and Clarifying them Through Examples
There are some misconceptions about the median, which we need to clarify to avoid any confusion. Here are some of the most common misconceptions along with examples:
A. It’s always a value from the data set: The median is not always a value in the dataset. For example, consider a set of numbers: 1, 1, 2, 3, 4. The median is 2, which is not one of the values in the dataset.
B. It’s always the same as the mode: The median and mode are not always the same. For example, consider a dataset of numbers: 1, 2, 3, 3, 4. In this case, the median is 3, and the mode is 3, but that isn’t always the case.
VII. How Median Can Be Used to Detect Outliers in a Data Set
An outlier is an observation in a dataset that significantly differs from the other observations. Median can be used to identify outliers in a data set with a box plot. In a box plot, the median is represented by the line splitting the box, and any data point outside the “whiskers” of the box (1.5 times the interquartile range) is considered an outlier.
VIII. Conclusion
Calculating the median is an essential part of data analysis, and it’s crucial to understand what it tells us about the data. The median is useful when dealing with skewed data with outliers as it is less vulnerable to extreme values than the mean. This article has covered a beginner’s guide to understanding the median, its calculation, real-life applications, comparison with other measures of central tendency, common misconceptions, and using it to detect outliers. We hope this article helps you use the median wisely for your data analysis, and remember to always interpret it by considering the context of your data.