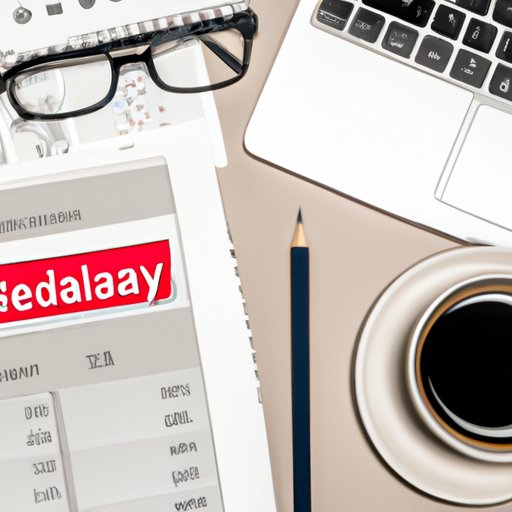
Introduction
Welcome to this article on residual calculation, a vital element of data analysis and business forecasting. As you delve into this article, you’ll get a clear understanding of residual calculation and its importance in real-life scenarios. You will learn how to calculate residuals, common mistakes to avoid, and alternative residual calculation methods which can give you a competitive edge.
Simple Explanation of Residual Calculation Using an Example
Residuals refer to the difference between an observed value in a dataset and the predicted value from a model. Residual calculation helps to determine how far calculated values are from actual values. Here’s how to calculate residuals:
Assume a regression model that predicts students’ exam scores. We have the following data set:
Scores: 60, 70, 80, 90, 100
Respective fitted values: 66, 75, 84, 93, 102
The residual for the first student’s score is obtained by:
Residual = Observed value – Fitted Value = 60 – 66 = -6
Therefore, the residual for the first student is -6. Using this same formula, we can compute the residual for each student in the dataset and summarize:
Residuals: -6, -5, -4, -3, -2
Importance of Residual in Data Analysis
Residual calculation is essential in data analysis. For instance, residual plots help us identify patterns in the data that may be missed by fitting methods. Data analysts use residual analysis to check for the adequacy of regression models and detect outliers. Poor residuals can also indicate poor model fit.
Residual calculation plays a critical role in model building, prediction accuracy, and model validation. When building regression models, residual analysis helps to identify which variables to include in the model accurately. Residual analysis plays a critical role in validating the prediction accuracy of a model in that high residuals show that the model is not making accurate predictions, and this needs to be corrected. Residual analysis provides a measure of model robustness. It helps in performing cross-validation checks to ensure that models’ accuracy is not due to overfitting.
Common Mistakes When Calculating Residual
Despite the importance of residual calculations in data analysis, errors can occur while performing residual calculations. A common mistake is failing to account for model flaws. Using a flawed model leads to inaccurate predictions and thus false residual values.
Another mistake is the omission of predictors that should be included in the model. This omission affects residual values since it neglects some of the variation between the model and actual values, leading to poor predictive power. A failure to remove outliers also leads to poor residual analyses.
To avoid these mistakes, ensure that the data used in modeling is appropriate for modeling purposes. Remove outliers and check for correlations between model coefficients.
Alternative Residual Calculation Methods
There are several approaches of calculating residuals. The traditional method of residual calculation is via least squares, but there exist other methods such as:
- Maximum likelihood estimation
- Generalized least squares
- Weighted least squares
Maximum likelihood estimation is a method that estimates the model parameters that maximize the partial likelihood function based on the data set. It assumes that the data is independently normally distributed around the mean and that the model residuals are also independent and normally distributed. Generalized least squares assume that the model residuals are correlated while weighted least squares account for non-constant variance across cases.
Each method has its strengths and weaknesses and can be applied in different scenarios.
The Relevance of Residual in Business Forecasting
The relevance of residual calculation in business forecasting cannot be understated. In business, residual calculation helps professionals to forecast crucial aspects such as sales, finance, inventory, and customer behavior among others. Residual analysis helps to identify data patterns, which are useful in modeling predictive systems. These systems provide managers with vital information that they use to make critical decisions at the organizational level. Residual analysis is applicable in different fields like retail, finance, healthcare, and marketing.
For instance, assume that a shoe retail business wants to determine its inventory levels. Residual analysis provides insights that help forecast inventory trends, making it easy for the business to make informed decisions on what to stock.
Conclusion
The importance of residual analysis in data analysis and business forecasting cannot be overstressed. By understanding how residual calculation works, you can avoid making common mistakes when performing residual calculations, use alternative calculation methods, and unlock new avenues for generating insights that you can apply to decision making. We hope that this article has provided you with a head start on residual analysis and that you can apply these principles in your future endeavors.