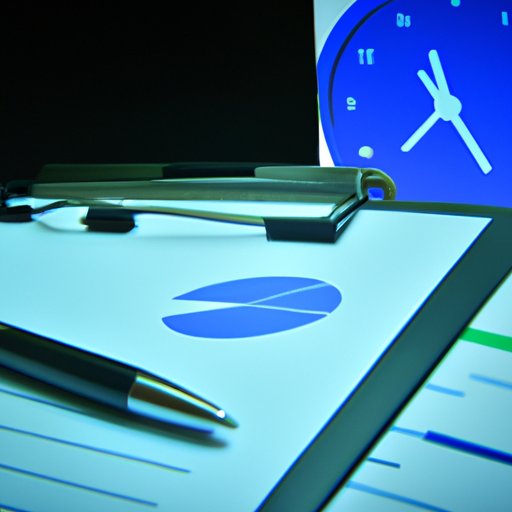
Introduction
When it comes to healthcare, data is everything. It is the backbone of decision-making, patient care, and operations. However, healthcare data can be unstructured, messy, and inconsistent, causing complicated issues. That’s where data normalization comes into play. Data normalization is the process of transforming raw data into consistent and standard values to improve data accuracy, interoperability, and decision-making. In this article, we will explore the importance of data normalization in healthcare, the techniques and strategies for normalizing data, and the benefits and challenges of this process.
Importance of Data Normalization in Healthcare: A Beginner’s Guide
Data normalization is critical to healthcare because it enables informed decision-making and improves patient outcomes. With normalized data, healthcare providers can easily compare, analyze, and share patient health information without encountering unexpected errors or disparities. It can also enhance the accuracy of medical claims, support population health management, and reduce the risk of fraud and abuse. Normalization can have a significant impact in healthcare scenarios such as clinical trials, medication management, and patient analytics.
The Ultimate Guide to Normalizing Data in Electronic Health Records (EHR)
Electronic Health Records (EHRs) are widely used in healthcare to store, retrieve, and share patient data efficiently. However, EHRs contain large amounts of unstandardized and unstructured data, which can affect interoperability and data accuracy. Normalizing data in EHRs involves several steps, such as data cleaning, standardization, and mapping. Data cleaning helps identify errors, inconsistencies, and redundancies. Standardization involves converting values into standard units and formats. Mapping aligns data points across different data sources. Best practices to maintain normalized data in EHRs include using a common data model, regular data quality checks, and prioritizing data governance.
Benefits and Challenges of Normalizing Healthcare Data: Insights for Healthcare Providers
The normalization of healthcare data presents several benefits for healthcare providers. It improves patient outcomes by making more informed clinical decision-making. It increases efficiency by reducing duplicative or unnecessary testing. Normalized data also allows for better benchmarking and quality reporting. However, normalization can also introduce some challenges. For instance, there may be variability in data across the organization or lack of standardized terminologies. Providers may also face challenges in comparing and mapping data from different electronic systems. To overcome these challenges, providers can focus on data governance, adopt clinical data standards, use appropriate data models, and engage in regular quality checks.
Techniques for Normalization of Clinical Data in Healthcare: A Review
Clinical data normalization is an essential part of healthcare data normalization. Concept normalization is a technique that maps synonymous concepts to a single term. Value set mapping involves aligning value sets with standards, such as ICD codes, to harmonize data. Other techniques include data element mapping, attribute mapping, and codification mapping. Each technique has its advantages and disadvantages, and providers need to choose the appropriate technique based on their specific needs.
Normalizing Healthcare Data for Better Quality Measures: An Overview
Normative healthcare data is essential to enable quality measurement programs. It allows healthcare organizations to establish benchmarks, identify areas of improvement, and report on quality initiatives. However, normalizing healthcare data for quality measures can be challenging due to the varying data sets and definitions used for quality reporting. Providers can overcome these challenges by adopting specific data standards, prioritizing data governance, and ensuring regular data quality checks.
Strategies for Normalizing Data in Healthcare Analytics: Best Practices and Tools
Healthcare analytics relies heavily on normalized data to generate insights and trends. One of the most critical strategies for normalizing data in healthcare analytics is through data mining, or identifying patterns and relationships in large data sets. Other strategies include data extrapolation, data sampling, and statistical analysis. The tools used for normalizing data in healthcare analytics include those for data cleansing, transformation, and mapping. These tools can be integrated into a larger data management system, or healthcare providers can opt for off-the-shelf enterprise software.
Conclusion
Data normalization is essential in healthcare because of its far-reaching impact on decision-making, patient outcomes, and efficiency. Providers and healthcare organizations must prioritize data governance, rely on appropriate techniques and strategies, and use reliable tools to normalize healthcare data. Accurate, complete, and normalized data is fundamental to deliver quality care and generate valuable insights into population health. With data normalization, healthcare providers can unlock the full potential of data to improve patient health outcomes and streamline operations.